For successful AI trading, it is important to pay attention to the management of risk. This is especially important when dealing with high-risk stocks such as the penny stocks or cryptos. Here are ten top tips on how you can incorporate the most effective risk management strategies into your AI trading strategy:
1. Define Risk Tolerance
Tip. Determine in writing the maximum loss that is acceptable for each trade, for daily drawsdowns, or for overall portfolio losses.
The AI trading system will be more precise if you know your risk tolerance.
2. Automated stop-loss and take-profit orders
Tip: Use AI to dynamically adjust stop-loss levels as well as take-profit levels according to the market's volatility.
Why: Automated safety measures limit losses and lock-in profits with no emotional involvement.
3. Diversify Your Portfolio
Tips: Spread your investment across different assets, sectors and markets (e.g., mix penny stocks, stocks with a large capital, and copyright).
Why: Diversification reduces the exposure to a particular asset's risk, while making sure that potential gains and losses are balanced.
4. Set Position Sizing Rules
Use AI to determine the size of your position based on:
Portfolio size.
Risk per trade (1-2 1 % of the portfolio value)
Asset volatility.
The reason: Proper sizing of your position will prevent overexposure to high-risk transactions.
5. Check for fluctuations and adjust strategies
Utilize indicators to gauge fluctuations, such as the VIX in stocks or on-chain information for copyright.
Why: Increased volatility requires more aggressive risk management and a flexible trading strategy.
6. Backtest Risk Management Rules
Tip Include risk-management parameters, such as stop loss levels and position sizing, when testing backtests to determine their efficacy.
Why testing is crucial to make sure that your risk management measures function in different market conditions.
7. Implement Risk-Reward Ratios
Tip - Make sure that every trade is based upon the risk/reward ratio 1:3 or greater (risking $1 to make $3).
What's the reason? Consistently using favorable ratios can improve long-term profitability, despite the occasional loss.
8. AI to detect and respond to anomalies
Create software for anomaly detection to spot unusual patterns in trading.
The importance of early detection is that it gives you time to make adjustments or end your positions prior to significant market movement.
9. Hedging Strategies for a Better investment
Tips: Make use of hedging strategies such as options or futures to reduce the risk.
Penny stocks can be hedged with ETFs that are in the same industry or comparable assets.
copyright: hedging using stablecoins and ETFs that are inverse.
Why is it important: Hedging guards against adverse price movements.
10. Regularly Monitor Risk Parameters and Adjust Them
As market conditions change it is important to review and adjust the risk settings of your AI trading system.
Why is this: a dynamic risk management ensures your plan is effective across different market conditions.
Bonus: Use Risk Assessment Metrics
Tip: Evaluate your strategy using metrics like:
Maximum Drawdown: The biggest portfolio decline from peak-to-trough.
Sharpe Ratio: Risk-adjusted return.
Win-Loss ratio: Number of profitable transactions versus losses.
The reason: These indicators offer insight into the performance of your strategy and the risk you are taking.
With these suggestions, it is possible to build a robust system for managing risk that will improve the efficiency and security of your AI-based trading strategies in penny stocks as well as copyright markets. Follow the best ai investing url for blog tips including best ai copyright, ai copyright trading bot, ai trade, ai for copyright trading, ai stock trading, stocks ai, using ai to trade stocks, ai predictor, ai trading bot, ai financial advisor and more.
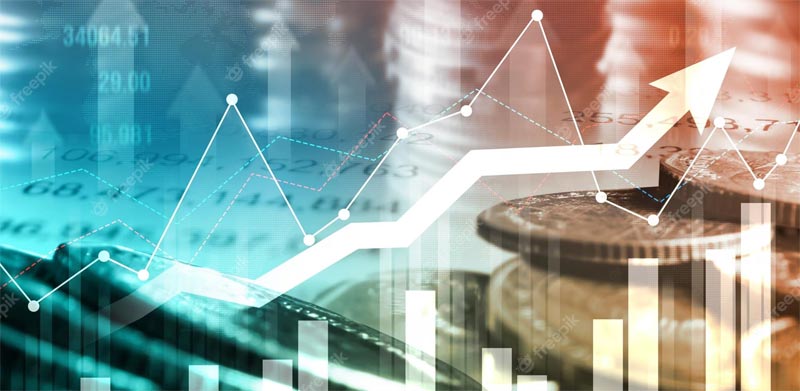
Top 10 Tips For Utilizing Ai Stock Pickers, Predictions, And Investments
To improve AI stockpickers and to improve investment strategies, it's crucial to make the most of backtesting. Backtesting can provide insight into the performance of an AI-driven strategy under past market conditions. These are 10 tips for using backtesting using AI predictions as well as stock pickers, investments and other investment.
1. Make use of high-quality historical data
Tip: Ensure the backtesting software uses complete and accurate historical data, including stock prices, trading volumes dividends, earnings reports, dividends as well as macroeconomic indicators.
The reason: High-quality data guarantees that backtesting results reflect realistic market conditions. Uncomplete or incorrect data can result in results from backtests being inaccurate, which could affect the reliability of your plan.
2. Incorporate real-time trading costs and Slippage
Backtesting is a great way to test the real-world effects of trading like transaction fees as well as slippage, commissions, and the impact of market fluctuations.
The reason: Not accounting for slippage and trading costs could result in overestimating the potential gains of your AI model. Incorporate these elements to ensure that your backtest is more realistic to the actual trading scenario.
3. Test different market conditions
Tip: Test your AI stock picker under a variety of market conditions, including bull markets, times of high volatility, financial crises or market corrections.
Why: AI algorithms may be different under different market conditions. Testing under various conditions can help ensure your strategy is flexible and durable.
4. Utilize Walk-Forward testing
Tips: Conduct walk-forward tests, where you compare the model to a rolling sample of historical data before confirming its performance with data from outside of your sample.
What is the reason? Walk-forward tests can help test the predictive power of AI models based upon untested evidence. It is an more accurate gauge of the performance of AI models in real-world conditions than static backtesting.
5. Ensure Proper Overfitting Prevention
Do not overfit the model through testing it using different time frames. Also, make sure the model does not learn the source of noise or anomalies from historical data.
The reason is that if the model is too tightly tailored to historical data, it becomes less accurate in predicting future movements of the market. A balanced, multi-market model must be generalizable.
6. Optimize Parameters During Backtesting
Utilize backtesting software to improve parameters like stop-loss thresholds and moving averages, or the size of your position by making adjustments incrementally.
Why: Optimising these parameters will improve the efficiency of AI. As mentioned previously, it's crucial to ensure that the optimization does not result in an overfitting.
7. Drawdown Analysis and Risk Management: Integrate Both
Tips: Use risk management techniques like stop-losses and risk-to-reward ratios and sizing of positions during backtesting to assess the strategy's ability to withstand large drawdowns.
The reason is that effective risk management is key to ensuring long-term financial success. Through simulating risk management within your AI models, you'll be capable of identifying potential weaknesses. This enables you to alter the strategy and get greater results.
8. Examine Key Metrics Other Than Returns
The Sharpe ratio is a key performance metric that goes far beyond simple returns.
What are these metrics? They will give you a more precise picture of the returns of your AI's risk adjusted. If you solely rely on returns, you could miss periods of high volatility or high risk.
9. Simulate Different Asset Classes & Strategies
TIP: Test your AI model using a variety of asset classes, such as stocks, ETFs or cryptocurrencies, and various strategies for investing, such as the mean-reversion investment, value investing, momentum investing and so on.
Why: Diversifying backtests across different asset classes lets you to test the flexibility of your AI model. This ensures that it is able to be utilized in multiple markets and investment styles. It also assists in making the AI model be effective when it comes to high-risk investments such as cryptocurrencies.
10. Regularly Update and Refine Your Backtesting Methodology
TIP: Always upgrade your backtesting system with the latest market information, ensuring it evolves to keep up with changing market conditions and new AI models.
The reason is because markets are constantly changing and so is your backtesting. Regular updates make sure that your AI models and backtests remain efficient, regardless of any new market or data.
Bonus Monte Carlo simulations may be used to assess risk
Tips: Use Monte Carlo simulations to model a wide range of outcomes that could be possible by performing multiple simulations using various input scenarios.
Why is that? Monte Carlo simulations are a fantastic way to determine the probability of a range of scenarios. They also offer an in-depth understanding of risk especially in markets that are volatile.
These suggestions will allow you to optimize and assess your AI stock picker by using tools for backtesting. An extensive backtesting process will guarantee that your AI-driven investments strategies are dependable, flexible and reliable. This lets you make informed decisions on volatile markets. Take a look at the top rated a replacement on stock ai for more advice including stocks ai, ai investing app, ai trader, ai in stock market, trading bots for stocks, trading with ai, ai stocks, ai for stock market, ai in stock market, ai trade and more.
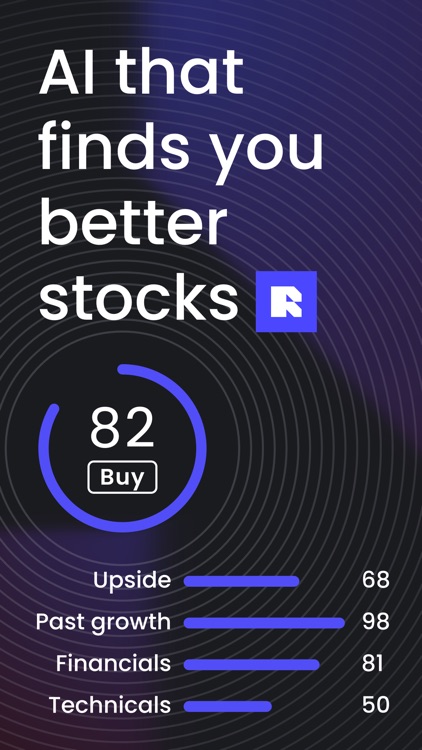